Backpropagation Program Directv
Computer-enhanced artificial intelligence (AI) has been around since the 1950s, but recent hardware innovations have reinvigorated the field. New sensors help machines have more accurate sight, hear sounds, and understand location. Powerful processors can help computers make complex decisions, sort through possibilities, plan outcomes, and learn from mistakes. The possibilities are thrilling; the implications are vast.This course will introduce you to some of the key concepts behind artificial intelligence, including the differences between 'strong' and 'weak' AI. You'll see how AI has created questions around what it means to be intelligent and how much trust we should put in machines. Instructor Doug Rose explains the different approaches to AI, including machine learning and deep learning, and the practical uses for new AI-enhanced technologies. Plus, learn how to integrate AI with other technology, such as big data, and avoid some common pitfalls associated with programming AI.
BackgroundBackpropagation is a common method for training a neural network. There is online that attempt to explain how backpropagation works, but few that include an example with actual numbers. This post is my attempt to explain how it works with a concrete example that folks can compare their own calculations to in order to ensure they understand backpropagation correctly.If this kind of thing interests you, you should where I post about AI-related projects that I’m working on. Backpropagation in PythonYou can play around with a Python script that I wrote that implements the backpropagation algorithm in. Backpropagation VisualizationFor an interactive visualization showing a neural network as it learns, check out my.
Additional ResourcesIf you find this tutorial useful and want to continue learning about neural networks, machine learning, and deep learning, I highly recommend checking out Adrian Rosebrock’s new book,. I really enjoyed the book and will have a full review up soon.
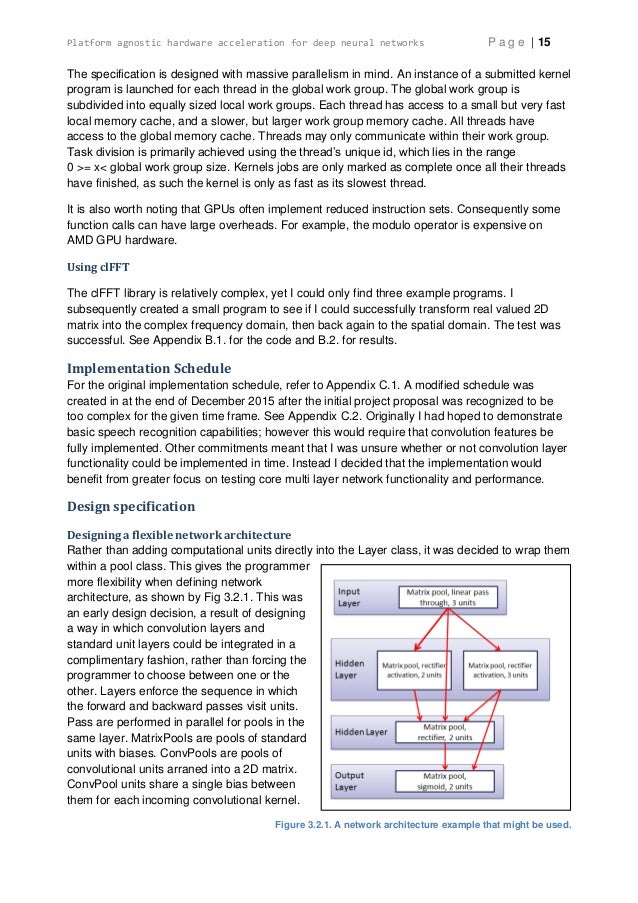
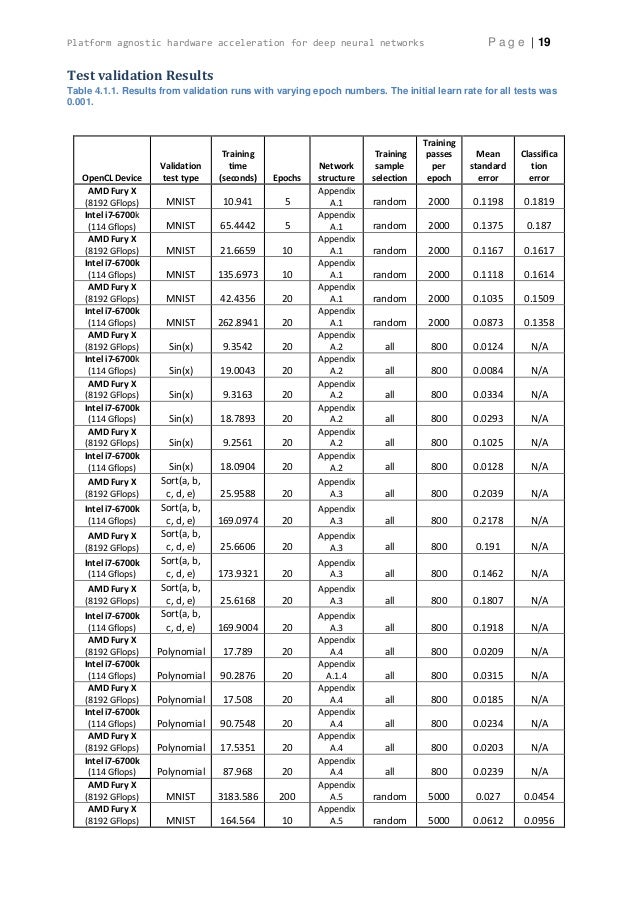
OverviewFor this tutorial, we’re going to use a neural network with two inputs, two hidden neurons, two output neurons. Additionally, the hidden and output neurons will include a bias.Here’s the basic structure:In order to have some numbers to work with, here are the initial weights, the biases, and training inputs/outputs:The goal of backpropagation is to optimize the weights so that the neural network can learn how to correctly map arbitrary inputs to outputs.For the rest of this tutorial we’re going to work with a single training set: given inputs 0.05 and 0.10, we want the neural network to output 0.01 and 0.99. The Forward PassTo begin, lets see what the neural network currently predicts given the weights and biases above and inputs of 0.05 and 0.10. To do this we’ll feed those inputs forward though the network.We figure out the total net input to each hidden layer neuron, squash the total net input using an activation function (here we use the logistic function), then repeat the process with the output layer neurons. Total net input is also referred to as just net input by.Here’s how we calculate the total net input for:We then squash it using the logistic function to get the output of:Carrying out the same process for we get:We repeat this process for the output layer neurons, using the output from the hidden layer neurons as inputs.Here’s the output for:And carrying out the same process for we get:Calculating the Total ErrorWe can now calculate the error for each output neuron using the and sum them to get the total error. The is included so that exponent is cancelled when we differentiate later on.
Register
Use (alpha) to represent the learning rate, (eta), and even use (epsilon).We can repeat this process to get the new weights, and:We perform the actual updates in the neural network after we have the new weights leading into the hidden layer neurons (ie, we use the original weights, not the updated weights, when we continue the backpropagation algorithm below). Hidden LayerNext, we’ll continue the backwards pass by calculating new values for, and.Big picture, here’s what we need to figure out:Visually:We’re going to use a similar process as we did for the output layer, but slightly different to account for the fact that the output of each hidden layer neuron contributes to the output (and therefore error) of multiple output neurons. We know that affects both and therefore the needs to take into consideration its effect on the both output neurons:Starting with:We can calculate using values we calculated earlier:And is equal to:Plugging them in:Following the same process for, we get:Therefore:Now that we have, we need to figure out and then for each weight:We calculate the partial derivative of the total net input to with respect to the same as we did for the output neuron:Putting it all together.
Watch Directv
You might also see this written as:We can now update:Repeating this for, andFinally, we’ve updated all of our weights! When we fed forward the 0.05 and 0.1 inputs originally, the error on the network was 0.298371109. After this first round of backpropagation, the total error is now down to 0.291027924. It might not seem like much, but after repeating this process 10,000 times, for example, the error plummets to 0. At this point, when we feed forward 0.05 and 0.1, the two outputs neurons generate 0.015912196 (vs 0.01 target) and 0.984065734 (vs 0.99 target).If you’ve made it this far and found any errors in any of the above or can think of any ways to make it clearer for future readers, don’t hesitate to.